In today’s fast-paced development environment, deploying and managing applications within Kubernetes clusters can be challenging. However, leveraging the right tools and workflows can simplify and automate this process, enhancing both efficiency and reliability. This article demonstrates how to deploy a WordPress application using GitHub, Helm charts, Argo CD, and Kubernetes, streamlining the deployment process and enabling developers to concentrate on creating exceptional software.
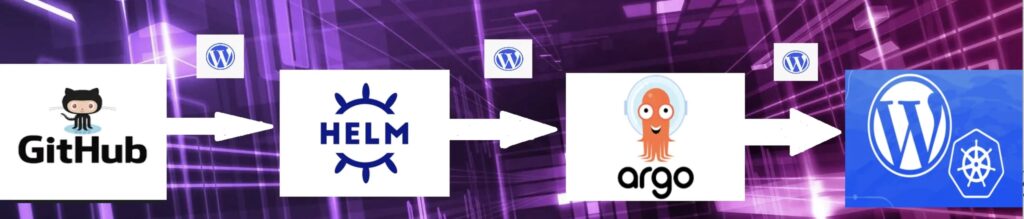
Setting Up the Environment
Before diving into the deployment process, it’s essential to set up the necessary tools and environments. This includes:
Setting Up GitHub Repository:
Create a GitHub repository to store Helm charts for both MySQL and WordPress applications. This repository will serve as the central source for version-controlled Helm charts.
Installing Helm:
Develop Helm charts for MySQL and WordPress applications needs Helm software. Helm charts define the structure and configuration of Kubernetes resources required to deploy the applications.
- Install
HELM
from this link : https://github.com/helm/helm/releases
Configuring Argo CD:
Install Argo CD, a GitOps continuous delivery tool, on your Kubernetes cluster. Configure Argo CD to sync with the GitHub repository containing the Helm charts.
- For installing
argo-cd
read this blog : https://harsh05.medium.com/streamlining-ci-cd-workflow-with-github-jenkins-sonarqube-docker-argocd-and-gitops-2563e0cbf7af
Helm Chart Development
1. MySQL Helm Chart:
Define the required Kubernetes resources for deploying a MySQL database, including Deployment, Service, and PersistentVolumeClaim (PVC). Configure parameters for customizing database settings such as username, password, and database name.
2. WordPress Helm Chart:
Develop a Helm chart for deploying the WordPress application, including Deployment, Service, and volume resources. Configure parameters for customizing WordPress settings such as site name, admin username, and password.
Next, let’s delve into the development of Helm charts for MySQL and WordPress applications:
- Clone your Github repository.
git clone <repository-link>

2. Create two folder inside it, one is mysql
and other is wordpress


3. Create Chart.yaml
file and templates
folder, values.yaml
file is optional
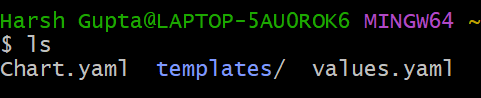
4. In chart file, copy below content :
apiVersion: v2
name: mysql
description: A Helm chart for Kubernetes
# A chart can be either an 'application' or a 'library' chart.
#
# Application charts are a collection of templates that can be packaged into versioned archives
# to be deployed.
#
# Library charts provide useful utilities or functions for the chart developer. They're included as
# a dependency of application charts to inject those utilities and functions into the rendering
# pipeline. Library charts do not define any templates and therefore cannot be deployed.
type: application
# This is the chart version. This version number should be incremented each time you make changes
# to the chart and its templates, including the app version.
# Versions are expected to follow Semantic Versioning (https://semver.org/)
version: 0.1.0
# This is the version number of the application being deployed. This version number should be
# incremented each time you make changes to the application. Versions are not expected to
# follow Semantic Versioning. They should reflect the version the application is using.
# It is recommended to use it with quotes.
appVersion: "1.16.0"
5. In templates folder, place all your manifests related to mysql, here we have three, deployment
, service
, volume
.
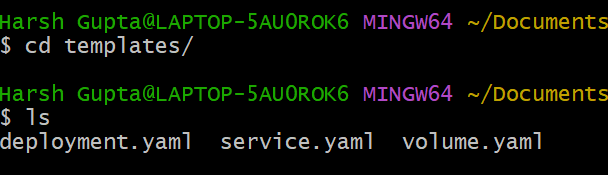
Content of the files :
# volume.yaml
apiVersion: v1
kind: PersistentVolumeClaim
metadata:
name: mysql-pv-claim
labels:
app: wordpress
spec:
accessModes:
- ReadWriteOnce
resources:
requests:
storage: 20Gi
# deployment.yaml
apiVersion: apps/v1
kind: Deployment
metadata:
name: wordpress-mysql
labels:
app: wordpress
spec:
selector:
matchLabels:
app: wordpress
tier: mysql
strategy:
type: Recreate
template:
metadata:
labels:
app: wordpress
tier: mysql
spec:
containers:
- image: mysql:latest
name: mysql
env:
- name: MYSQL_ROOT_PASSWORD
valueFrom:
secretKeyRef:
name: mysql-pass
key: password
- name: MYSQL_DATABASE
value: wordpress
- name: MYSQL_USER
value: wordpress
- name: MYSQL_PASSWORD
valueFrom:
secretKeyRef:
name: mysql-pass
key: password
ports:
- containerPort: 3306
name: mysql
volumeMounts:
- name: mysql-persistent-storage
mountPath: /var/lib/mysql
volumes:
- name: mysql-persistent-storage
persistentVolumeClaim:
claimName: mysql-pv-claim
# service.yaml
apiVersion: v1
kind: Service
metadata:
name: wordpress-mysql
labels:
app: wordpress
spec:
ports:
- port: 3306
selector:
app: wordpress
tier: mysql
clusterIP: None
6. In the same way, we will create wordpress
chart also.


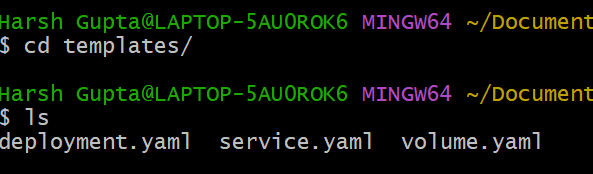
Content of the files :
# Chart.yaml
apiVersion: v2
name: wordpress
description: A Helm chart for Kubernetes
# A chart can be either an 'application' or a 'library' chart.
#
# Application charts are a collection of templates that can be packaged into versioned archives
# to be deployed.
#
# Library charts provide useful utilities or functions for the chart developer. They're included as
# a dependency of application charts to inject those utilities and functions into the rendering
# pipeline. Library charts do not define any templates and therefore cannot be deployed.
type: application
# This is the chart version. This version number should be incremented each time you make changes
# to the chart and its templates, including the app version.
# Versions are expected to follow Semantic Versioning (https://semver.org/)
version: 0.1.0
# This is the version number of the application being deployed. This version number should be
# incremented each time you make changes to the application. Versions are not expected to
# follow Semantic Versioning. They should reflect the version the application is using.
# It is recommended to use it with quotes.
appVersion: "1.16.0"
# deployment.yaml
apiVersion: apps/v1
kind: Deployment
metadata:
name: wordpress
labels:
app: wordpress
spec:
selector:
matchLabels:
app: wordpress
tier: frontend
strategy:
type: Recreate
template:
metadata:
labels:
app: wordpress
tier: frontend
spec:
containers:
- image: wordpress:latest
name: wordpress
env:
- name: WORDPRESS_DB_HOST
value: wordpress-mysql
- name: WORDPRESS_DB_PASSWORD
valueFrom:
secretKeyRef:
name: mysql-pass
key: password
- name: WORDPRESS_DB_USER
value: wordpress
ports:
- containerPort: 80
name: wordpress
volumeMounts:
- name: wordpress-persistent-storage
mountPath: /var/www/html
volumes:
- name: wordpress-persistent-storage
persistentVolumeClaim:
claimName: wp-pv-claim
# service.yaml
apiVersion: v1
kind: Service
metadata:
name: wordpress
labels:
app: wordpress
spec:
ports:
- port: 80
selector:
app: wordpress
tier: frontend
type: LoadBalancer
# volume.yaml
apiVersion: v1
kind: PersistentVolumeClaim
metadata:
name: wp-pv-claim
labels:
app: wordpress
spec:
accessModes:
- ReadWriteOnce
resources:
requests:
storage: 20Gi
6. Use the helm lint command to test the Helm chart locally:
helm.exe lint <dir-path>
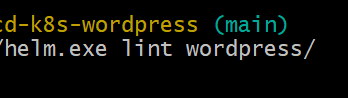

7. Add robots.txt at the root location of the repository. This will avoid bot crawling on the Helm repository.
echo -e “User-Agent: *\nDisallow: /” > robots.txt
8. Package your Helm chart
helm.exe package mysql/
helm.exe package wordpress/


This will create .tgz file at root location of the repository.

GitHub Repository Setup
Once the Helm charts are developed, it’s time to upload them to the GitHub repository:
- Creating Helm repository using github pages.

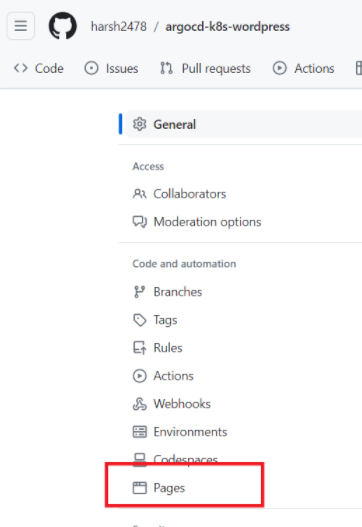
→ Select your branch in the Source section.
→ Click on Save.
→ You will find a link for your public Helm chart repository.
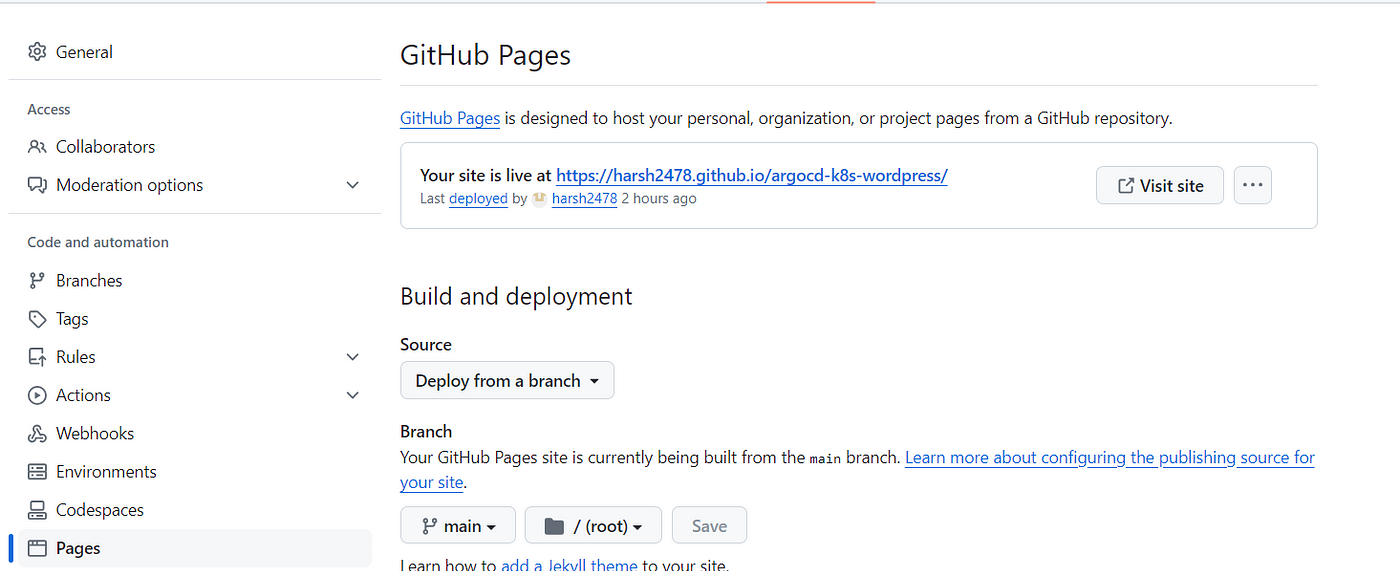
→ Click on the link and wait for some time to see your README.md contents on the screen, as shown below.
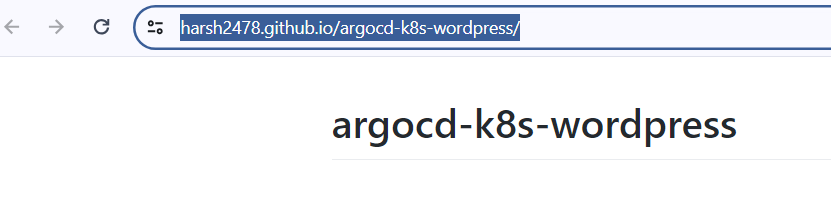
2. Creating index.yaml
file for Helm repository.
helm.exe repo index --url <repo-link> .

3. Pushing Helm Charts:
Add Helm chart directories to the GitHub repository and commit changes. Ensure that the directory structure follows the Helm chart conventions.
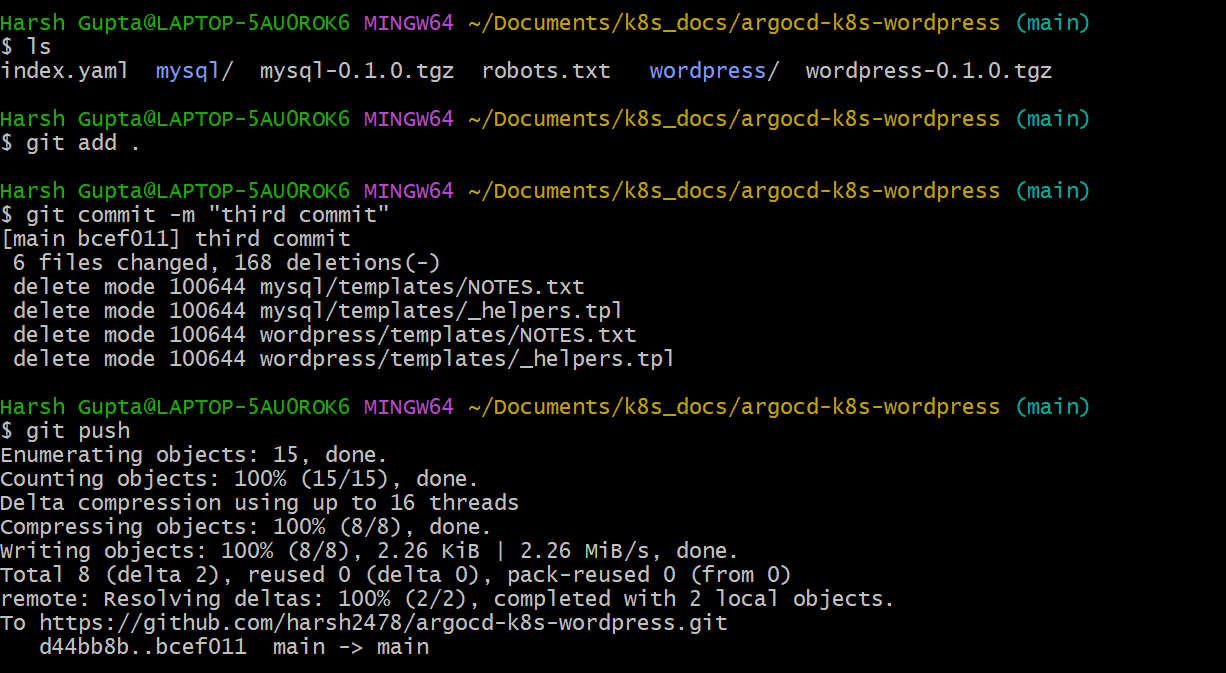
Argo CD Integration
Integrate the GitHub repository containing Helm charts with Argo CD for automated deployments:
1. Adding Helm Repository:
In the Argo CD UI, navigate to Settings > Repository and add the GitHub repository URL as a Helm repository. Configure synchronization settings to enable automatic syncing.
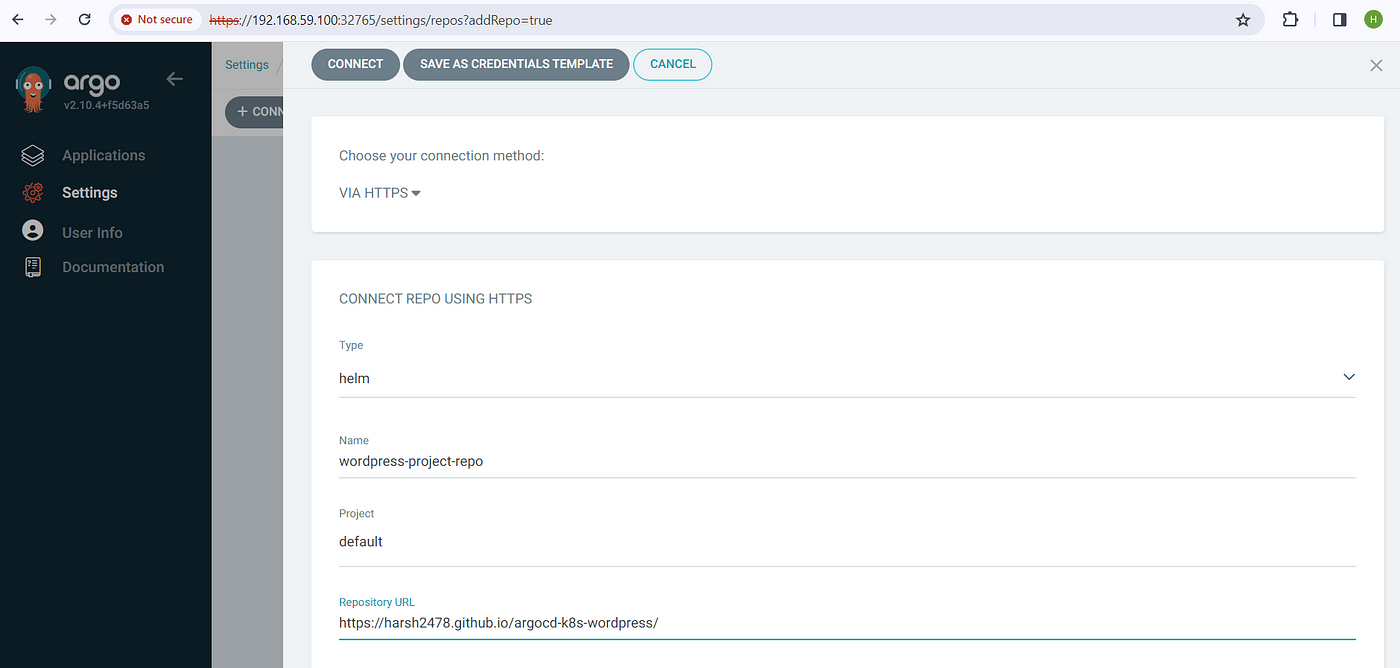

2. Creating Applications:
Create two new applications in Argo CD for MySQL and WordPress, specifying the Helm chart paths and version constraints. Argo CD will automatically detect changes in the Helm charts and initiate deployments.
MYSQL :

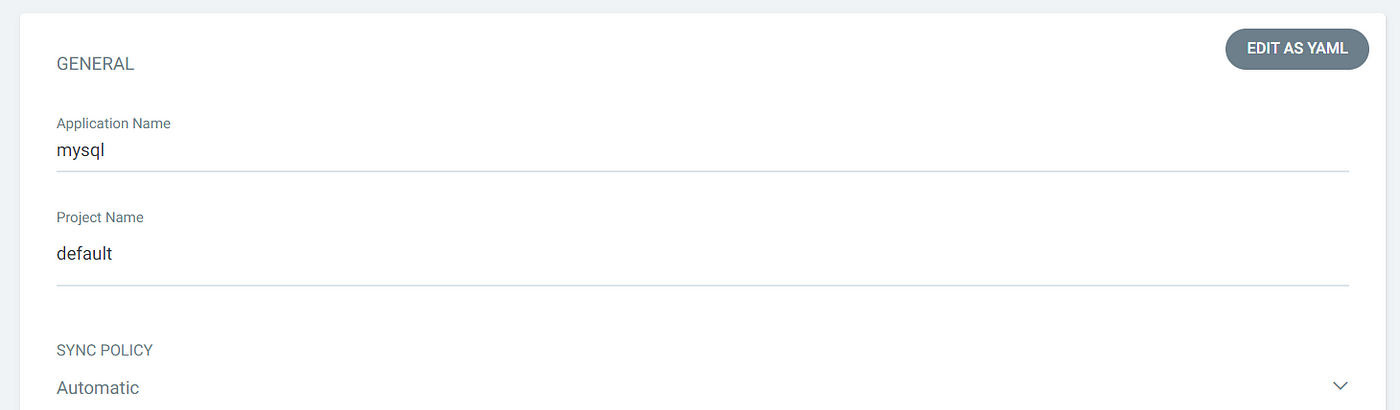
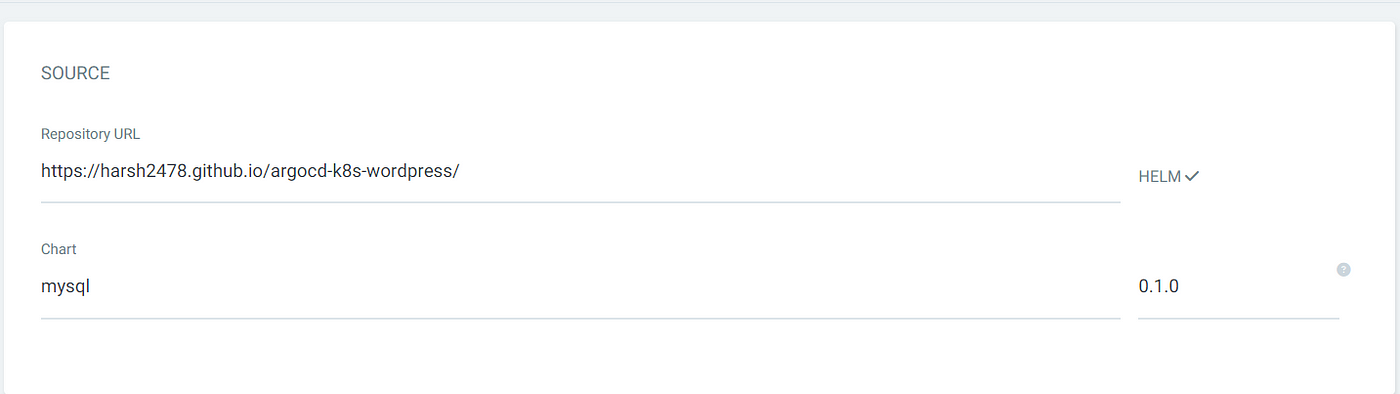
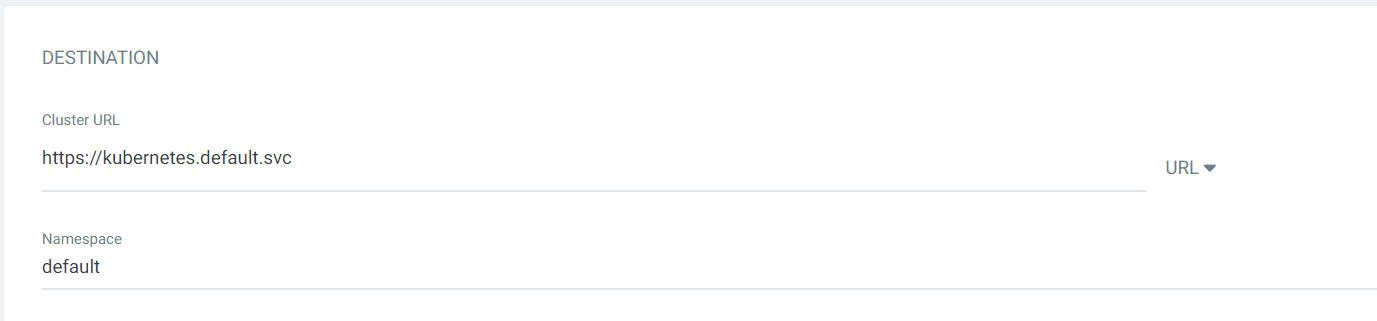
WORDPRESS :

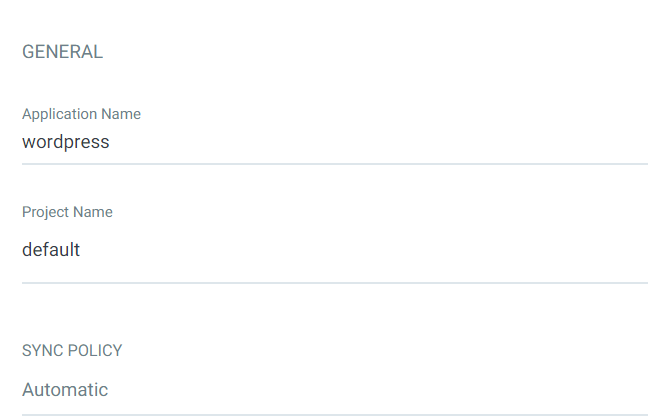
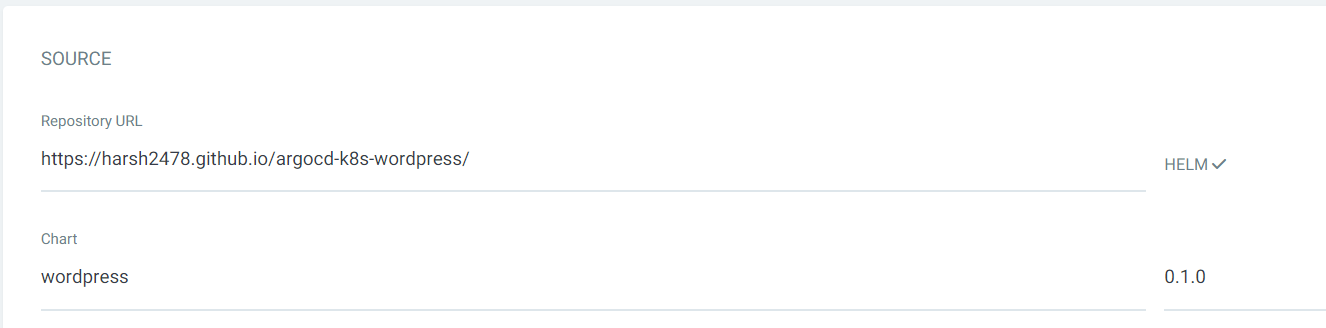
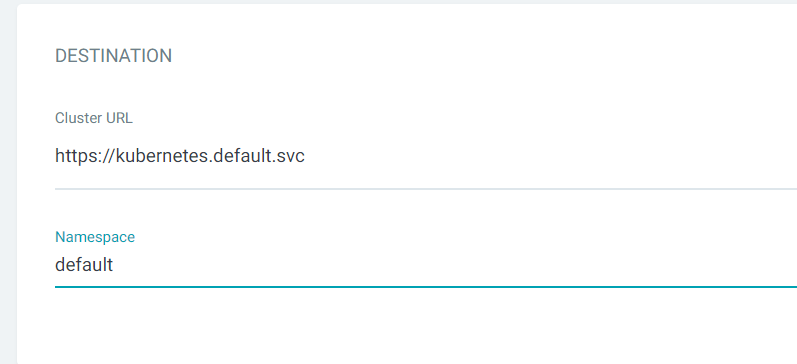
Deployment and Accessing WordPress
With the setup complete, Argo CD will automatically sync and deploy the MySQL and WordPress applications to the Kubernetes cluster:
Deployment Process:
Argo CD will pull the latest Helm charts from the GitHub repository, apply the desired configurations, and deploy the applications to the Kubernetes cluster.
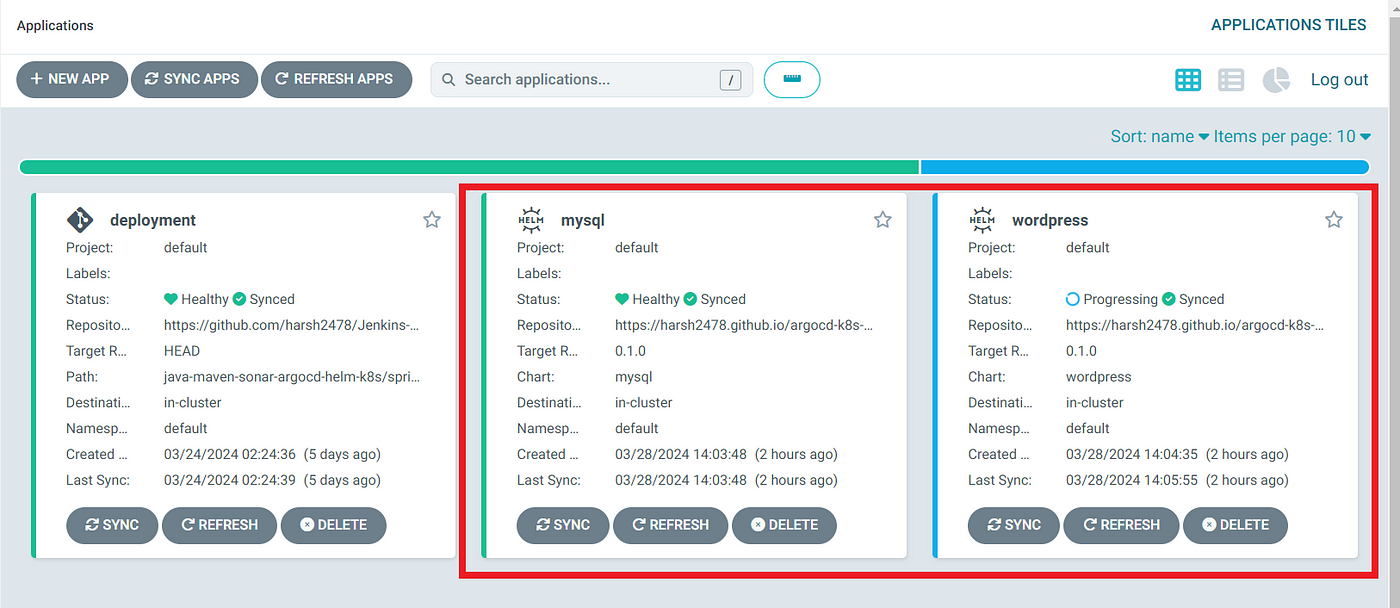
Accessing WordPress:
Once the deployments are complete, access the WordPress site by navigating to the specified Ingress URL. Log in using the provided credentials and start configuring your WordPress site.

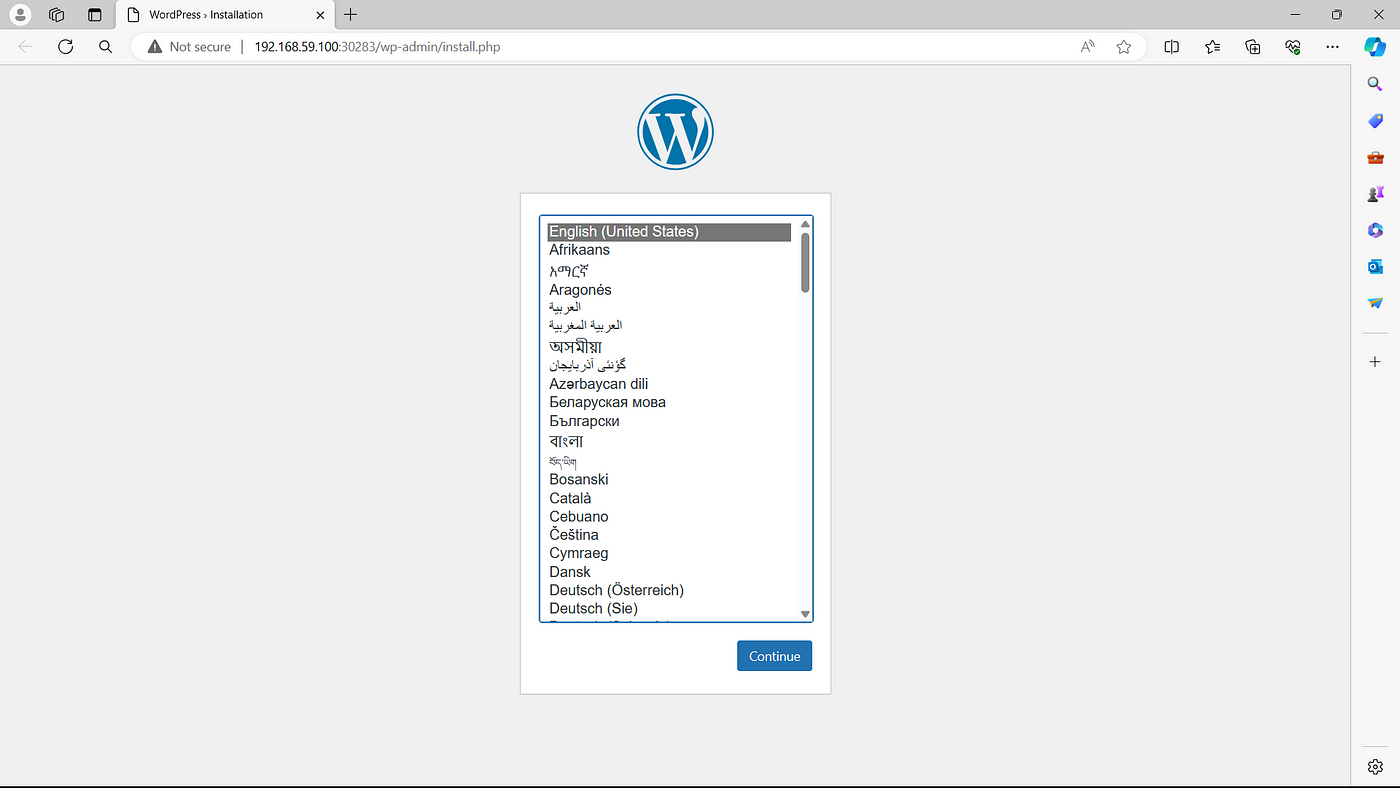
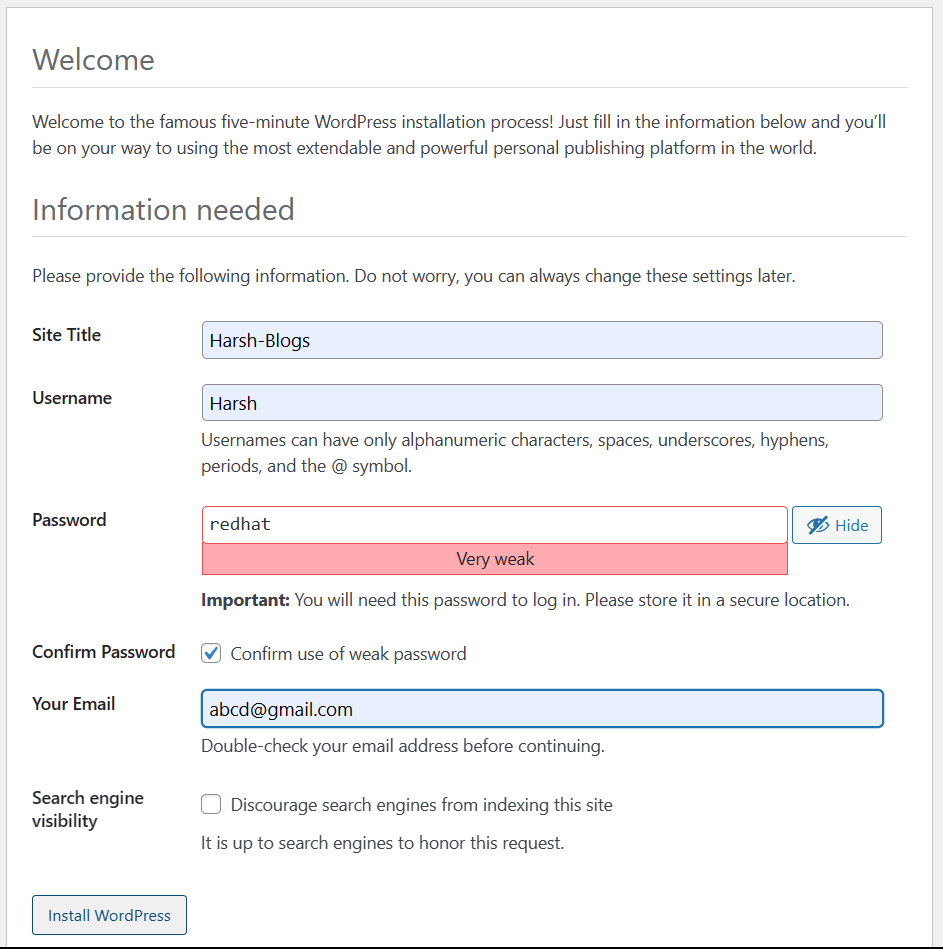
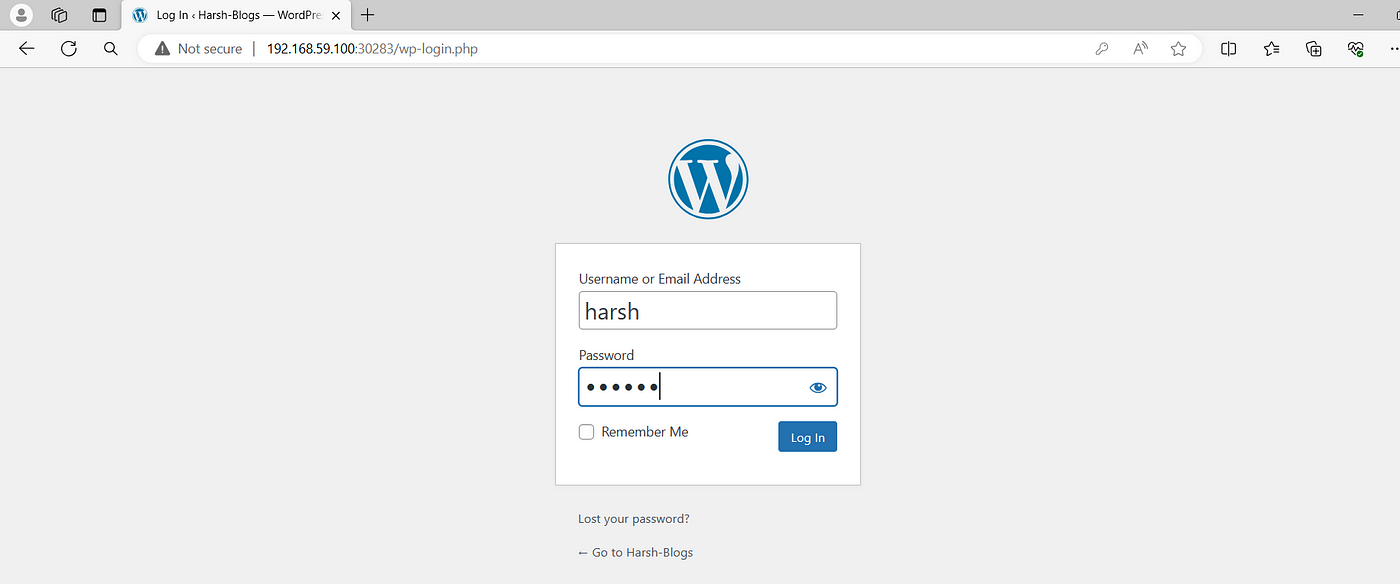
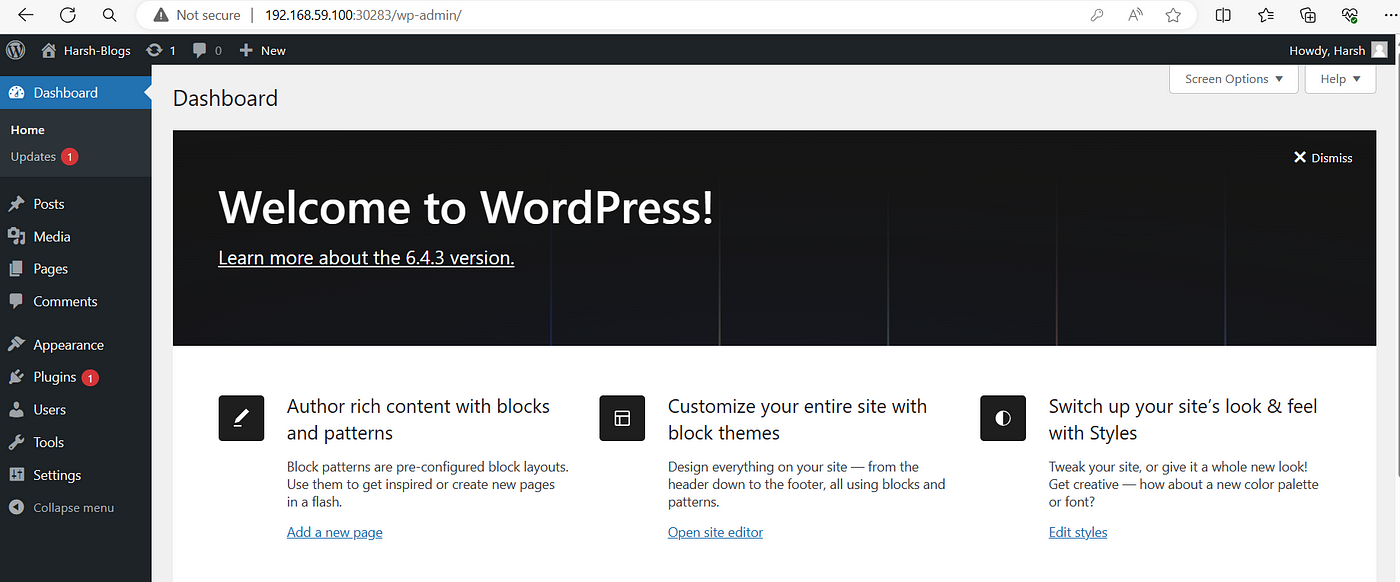